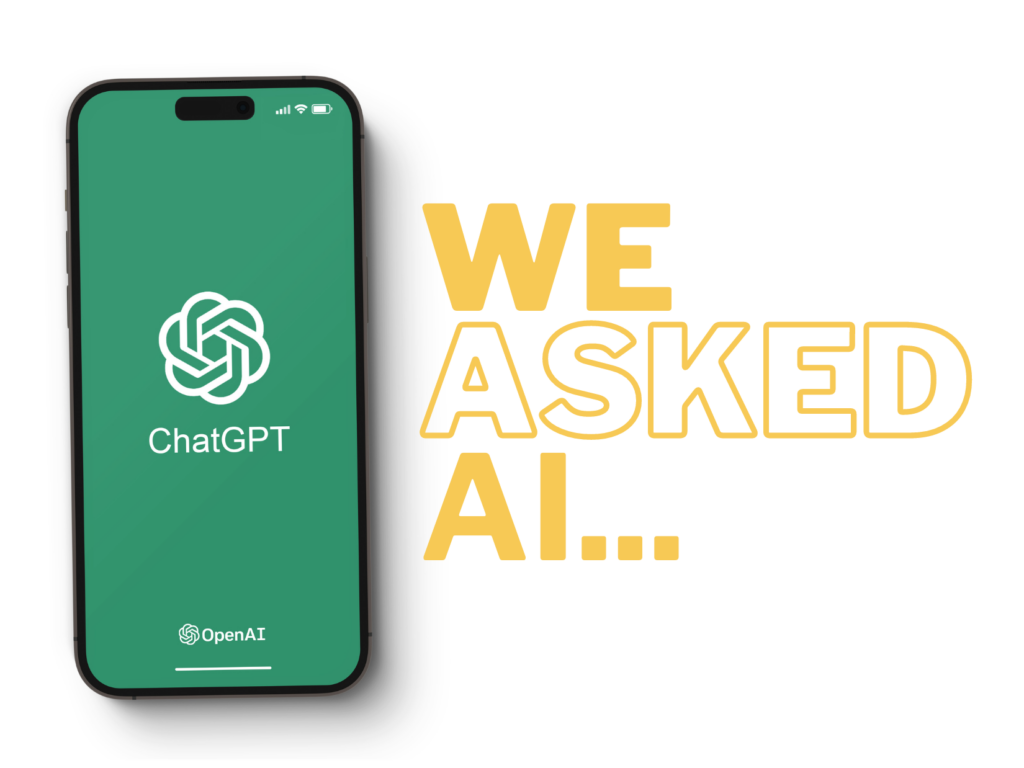
ChatGPT seems to have all the answers, so we decided to see how honest it would be about itself. Its answers were revealing. Join us for this ongoing blog series called “We Asked AI…” to learn how artificial intelligence in its various forms might perform when used for contract management.
The Human Prompt
What are 5 examples of ML failure in legal review that could have negative consequences for F500 companies?
The Unedited Answer from ChatGPT
Machine learning (ML) systems, including those used for legal review, are not immune to failures. Here are five examples of ML failures in legal review that could have negative consequences for Fortune 500 (F500) companies:
Bias in Training Data
- Failure Scenario: If the ML model is trained on biased or incomplete legal data, it can perpetuate and even amplify existing biases. For instance, if historical legal documents used for training disproportionately favor certain groups, the model may exhibit biased decision-making.
- Consequence: Legal decisions or recommendations may systematically favor or disadvantage certain demographics, potentially leading to legal challenges and damage to a company’s reputation.
Misinterpretation of Ambiguous Language
- Failure Scenario: ML models may struggle to accurately interpret ambiguous language or nuanced legal concepts. If the model is not trained to handle context well, it might misinterpret critical legal terms or miss important subtleties in legal documents.
- Consequence: Incorrect legal assessments could result in misguided decisions, leading to financial losses, contractual disputes, or regulatory non-compliance for the F500 company.
Inadequate Data Security
- Failure Scenario: ML models trained on legal documents may inadvertently expose sensitive information if not designed with robust data security measures. This could result in the unauthorized disclosure of confidential or proprietary information.
- Consequence: Legal breaches may lead to legal actions against the company, financial penalties, and reputational damage. Additionally, it may violate privacy regulations.
Lack of Explainability
- Failure Scenario: If an ML model lacks transparency and cannot provide clear explanations for its decisions, legal professionals may struggle to understand the rationale behind specific recommendations or rulings.
- Consequence: Legal decisions that cannot be adequately explained may be challenged, potentially leading to legal disputes and regulatory scrutiny. Lack of transparency may also hinder the company’s ability to comply with legal requirements.
Failure to Adapt to Regulatory Changes
- Failure Scenario: ML models may become outdated or non-compliant with evolving legal regulations if not regularly updated. Failure to adapt to changes in laws and regulations can result in the generation of inaccurate legal advice or non-compliance with new legal standards.
- Consequence: F500 companies relying on outdated legal advice may face legal consequences, financial penalties, and reputational harm. Non-compliance with current legal standards can have severe consequences for business operations.
It’s important for companies to be aware of the limitations and potential pitfalls of ML systems in legal review and to implement safeguards to mitigate these risks. Regular audits, transparency in model decision-making, and continuous monitoring and updates are essential components of a robust ML system in a legal context.
Human Note: Prompt generated on November 15, 2023, from ChapGPT 4.
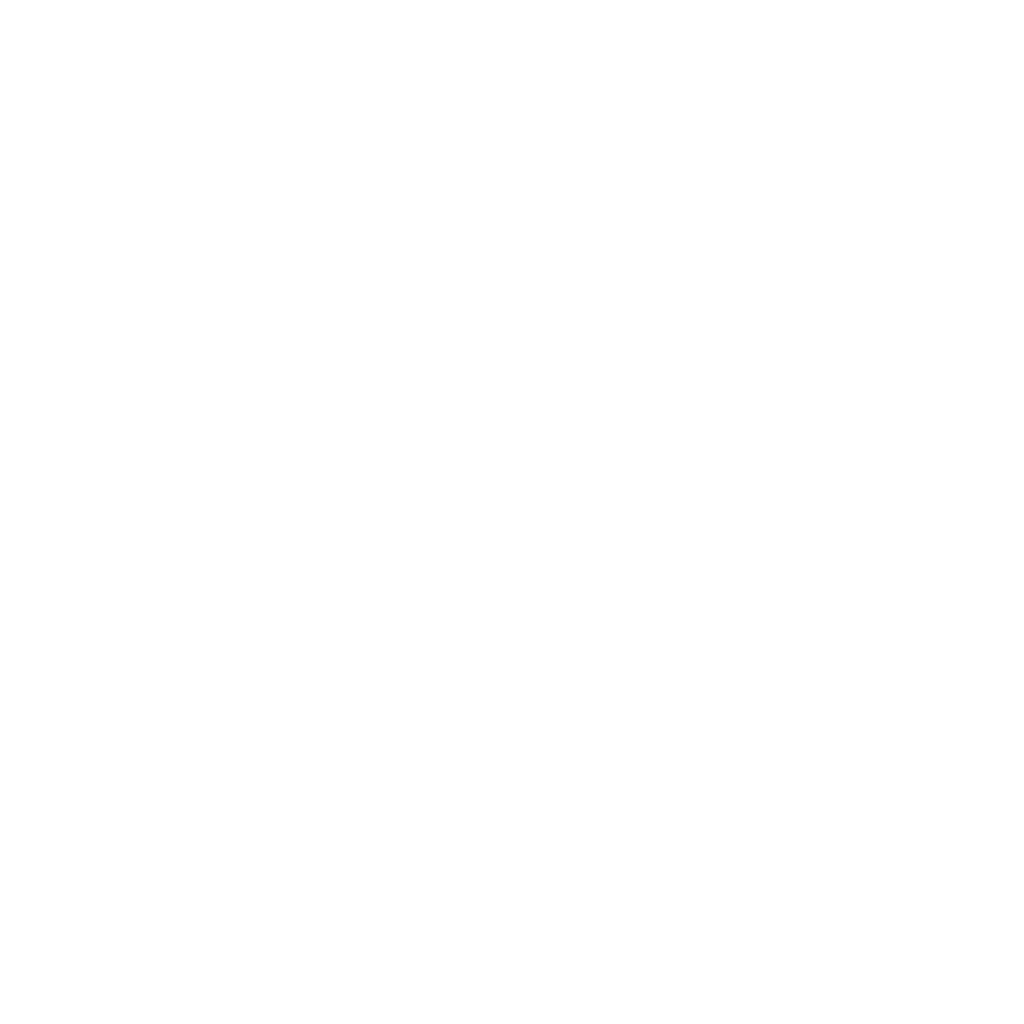
CLOC Ask The Experts: Is ChatGPT or Machine Learning the Right Tool for Analyzing Your Contracts?
Speakers from Cruise, Coinbase and Knowable